Integrating cognitive antecedents into TAM to explain mobile banking behavioral intention: A SEM-neural network modeling
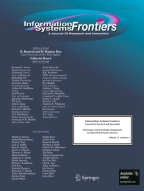
Higher penetration of smartphones and 3G and 4G mobile networks have led to the higher usage of smartphones for mobile banking activities. This paper identifies key antecedents influencing the mobile banking acceptance. The research extends the original Technology Acceptance Model, by incorporating two cognitive antecedents, namely, autonomous motivation and controlled motivation, in addition to trust components for understanding adoption. Data were collected from 225 mobile banking users and analyzed using an innovative two-stage research methodology. In the first stage, structural equation modeling was employed to test the research hypotheses and identify significant antecedents influencing mobile banking acceptance. In the second stage, the significant antecedents obtained from the first stage were input to a neural network model for ranking. The results showed that trust and autonomous motivation are the two main predictors influencing mobile banking acceptance. Theoretical and practical implications of findings are discussed.
This is a preview of subscription content, log in via an institution to check access.
Access this article
Subscribe and save
Springer+ Basic
€32.70 /Month
- Get 10 units per month
- Download Article/Chapter or eBook
- 1 Unit = 1 Article or 1 Chapter
- Cancel anytime
Buy Now
Price includes VAT (France)
Instant access to the full article PDF.
Rent this article via DeepDyve
Similar content being viewed by others
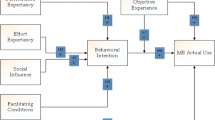
Self-Reported and Computer-Recorded Experience in Mobile Banking: a Multi-Phase Path Analytic Approach
Article 02 January 2019
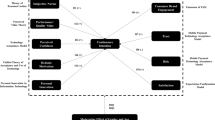
Examining the determinants of continuance intention to use and the moderating effect of the gender and age of users of NFC mobile payments: a multi-analytical approach
Article 20 April 2021
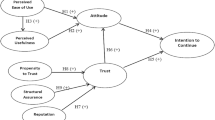
Customers’ Continuance Intention to Use Mobile Banking: Development and Testing of an Integrated Model
Article 04 March 2020
Explore related subjects
References
- Adams, D. A., Nelson, R. R., Todd, P., & A. (1992). Perceived usefulness, ease of use and usage of information technology: A replication. MIS Quarterly, 16(2), 227–247. ArticleGoogle Scholar
- Akturan, U., & Tezcan, N. (2012). Mobile banking adoption of the youth market. Marketing Intelligence & Planning, 30(4), 444–459. ArticleGoogle Scholar
- Alalwan, A. A., Dwivedi, Y. K., Rana, N. P., & Simintiras, A. C. (2016). Jordanian consumers' adoption of telebanking: Influence of perceived usefulness, trust, and self-efficacy. International Journal of Bank Marketing, 34(5), 690–709. ArticleGoogle Scholar
- Alalwan, A. A., Dwivedi, Y. K., & Rana, N. P. (2017). Factors influencing adoption of mobile banking by Jordanian bank customers: Extending UTAUT2 with trust. International Journal of Information Management, 37(3), 99–110. ArticleGoogle Scholar
- Barati, S., & Mohammadi, S. (2009). An efficient model to improve customer acceptance of mobile Banking. Proceedings of the world congress on engineering and computer Science, vol II WCECS, October 20–22, 2009, San Francisco.
- Bellenger, D. N., & Korgaonkar, P. (1980). Profiling the recreational shopper. Journal of Retailing, 56(3), 77–82. Google Scholar
- Bremer, C. (2012). New format for online courses: The open course future of learning. Proceedings of eLearning Baltics eLBa, 2012, 63–90. Google Scholar
- Brown, I., Cajee, Z., Davies, D., & Stroebel, S. (2003). Cell phone banking: Predictors of adoption in South Africa-an exploratory study. International Journal of Information Management, 23(5), 381–394. ArticleGoogle Scholar
- Chang, S. C., & Tung, F. C. (2008). An empirical investigation of students' behavioural intentions to use the online learning course websites. British Journal of Educational Technology, 39(1), 71–83. Google Scholar
- Chong, L.-Y. A. (2013). A two staged SEM-neural network approach for understanding and predicting the determinants of m-commerce adoption. Expert Systems with Applications, 40, 1240–1247. ArticleGoogle Scholar
- Chong, A. Y. L., Chan, F. T., & Ooi, K. B. (2012). Predicting consumer decisions to adopt mobile commerce: Cross country empirical examination between China and Malaysia. Decision Support Systems, 53(1), 34–43. ArticleGoogle Scholar
- Chong, A. Y. L., Liu, M. J., Luo, J., & Keng-Boon, O. (2015). Predicting RFID adoption in healthcare supply chain from the perspectives of users. International Journal of Production Economics, 159, 66–75. ArticleGoogle Scholar
- Dai, H., & Palvi, P. C. (2009). Mobile commerce adoption in China and the United States: A cross-cultural study. ACM SIGMIS Database, 40(4), 43–61. ArticleGoogle Scholar
- Davis, F. D. (1989). Perceived usefulness, perceived ease of use, and user acceptance of information technology. MIS Quarterly, 13(3), 319–340. ArticleGoogle Scholar
- Davis, F. D., Bagozzi, R. P., & Warshaw, P. R. (1989). User acceptance of computer technology: A comparison of two theoretical models. Management Science, 35, 982–1003. ArticleGoogle Scholar
- De Naeghel, J., Van Keer, H., Vansteenkiste, M., Haerens, L., & Aelterman, N. (2016). Promoting elementary school students' autonomous reading motivation: Effects of a teacher professional development workshop. The Journal of Educational Research, 109(3), 232–252. ArticleGoogle Scholar
- Deci, E. L., & Ryan, R. M. (2002). Handbook of self-determination research. University Rochester Press.
- Delone, W. H., & McLean, E. R. (2003). The DeLone and McLean model of information systems success: A ten-year update. Journal of Management Information Systems, 19(4), 9–30. ArticleGoogle Scholar
- Drewes, D. W. (2000). Beyond the spearman-Brown: A structural approach tomaximal reliability. Psychological Methods, 5, 214–227. ArticleGoogle Scholar
- Fishbein, M., & Ajzen, I. (1975). Belief, Attitude, Intention and Behavior: An introduction to Theory and Research. Addison-Wesley, Reading.
- Gagne, P., & Hancock, G. R. (2006). Measurement model quality, sample size, and solution propriety in confirmatory factor models. Multivariate Behavioral Research, 41(1), 65–83. ArticleGoogle Scholar
- Goodhue, D. L., & Thompson, R. L. (1995). Task-technology fit and individual performance. MIS Quarterly., 19, 213–236. ArticleGoogle Scholar
- Gu, J. C., Lee, S. C., & Suh, Y. H. (2009). Determinants of behavioral intention to mobile banking. Expert Systems with Applications, 36(9), 11605–11616. ArticleGoogle Scholar
- Guàrdia, L., Maina, M., & Sangrà, A. (2013). MOOC design principles: A pedagogical approach from the learner’s perspective. eLearning Papers, 33,1–6.
- Gulf News (2015). GCC Banks lag in Smart-Phone Banking and Digital Customer Experience. http://gulfnews.com/business/sectors/banking/gcc-banks-lag-in-smartphone-banking-and-digital-customer-experience-1.1620262. Accessed 15 Nov 2015.
- Hagger, M. S., Chatzisarantis, N. L., & Biddle, S. J. (2002). A meta-analytic review of the theories of reasoned action and planned behavior in physical activity: Predictive validity and the contribution of additional variables. Journal of Sport & Exercise Psychology, 24(1), 3–32. ArticleGoogle Scholar
- Hair, J. F., Anderson, R. E., Babin, B. J., & Black, W. C. (2010). Multivariate data analysis: A global perspective (Vol. 7). Upper Saddle River: Pearson. Google Scholar
- Hanafizadeh, P., Behboudi, M., Koshksaray, A. A., & Tabar, S. J. M. (2014). Mobile banking adoption by Iranian bank clients. Telematics & Informatics, 31, 62–78. ArticleGoogle Scholar
- Hartnett, M. K. (2015). Influences that undermine learners’ perceptions of autonomy, competence and relatedness in an online context. Australasian Journal of Educational Technology, 31(1), 86–99. ArticleGoogle Scholar
- Haykin, S. S. (2001). Neural networks: A comprehensive foundation. Beijing: Tsinghua University Press. Google Scholar
- Kapoor, K. K., Dwivedi, Y. K., & Williams, M. D. (2015). Examining the role of three sets of innovation attributes for determining adoption of the interbank mobile payment service. Information Systems Frontiers, 17(5), 1039–1056. ArticleGoogle Scholar
- Kim, H. W., Chan, H. C., & Gupta, S. (2007). Value-based adoption of mobile internet: An empirical investigation. Decision Support Systems, 43(1), 111–126. ArticleGoogle Scholar
- Laukkanen, T., & Kiviniemi, V. (2010). The role of information in mobile banking resistance. International Journal of Bank Marketing, 28(5), 372–388. ArticleGoogle Scholar
- Lee, Y., Lee, J., & Hwang, Y. (2015). Relating motivation to information and communication technology acceptance: Self-determination theory perspective. Computers in Human Behavior, 51, 418–428. ArticleGoogle Scholar
- Liébana-Cabanillas, F., Sánchez-Fernández, J., & Muñoz-Leiva, F. (2014). The moderating effect of experience in the adoption of mobile payment tools in virtual social networks: The m-payment acceptance model in virtual social networks (MPAM-VSN). International Journal of Information Management, 34(2), 151–166. ArticleGoogle Scholar
- Liébana-Cabanillas, F., Marinković, V., & Kalinić, Z. (2017). A SEM-neural network approach for predicting antecedents of m-commerce acceptance. International Journal of Information Management, 37(2), 14–24. ArticleGoogle Scholar
- Lin, F. H. (2011). An empirical investigation of mobile banking adoption: The effect of innovation attributes and knowledge based trust. International Journal of Information Management, 31, 252–260. ArticleGoogle Scholar
- Luarn, P., & Lin, H. H. (2005). Toward an understanding of the behavioral intention to use mobile banking. Computers in Human Behavior, 21(6), 873–891. ArticleGoogle Scholar
- Luo, X., Li, H., Zhang, J., & Shim, J. P. (2010). Examining multi-dimensional trust and multi-faceted risk in initial acceptance of emerging technologies: An empirical study of mobile banking services. Decision Support Systems, 49(2), 222–234. ArticleGoogle Scholar
- Malaquias, F. R., & Hwang, Y. (2016). An empirical study on trust in mobile banking: A developing country perspective. Computers in Human Behavior, 54, 453–461. ArticleGoogle Scholar
- Mallat, N., Rossi, M., Tuunainen, V. K., & Öörni, A. (2009). The impact of use context on mobile services acceptance: The case of mobile ticketing. Information Management, 46(3), 190–195. ArticleGoogle Scholar
- Michou, A., Matsagouras, E., & Lens, W. (2014). Dispositional achievement motives matter for autonomous versus controlled motivation and behavioral or affective educational outcomes. Personality and Individual Differences, 69, 205–211. ArticleGoogle Scholar
- Nikou, S. A., & Economides, A. A. (2017). Mobile-based assessment: Integrating acceptance and motivational factors into a combined model of self-determination theory and technology acceptance. Computers in Human Behavior, 68, 83–95. ArticleGoogle Scholar
- O’Connor, Y., & O’Reilly, P. (2016). Examining the infusion of mobile technology by healthcare practitioners in a hospital setting. Information Systems Frontiers, 1–21.
- Riffai, M. M. M. A., Grant, K., & Edgar, D. (2012). Big TAM in Oman: Exploring the promise of on-line banking, its adoption by customers and the challenges of banking in Oman. International Journal of Information Management, 32(3), 239–250.
- Ryan, R. M., & Deci, E. L. (2000). Intrinsic and extrinsic motivations: Classic definitions and new directions. Contemporary educational psychology, 25(1), 54–67.
- Ryan, R. M., & Deci, E. L. (2000). Self-determination theory and the facilitation of intrinsic motivation, social development, and well-being. American Psychologist, 55(1), 68. ArticleGoogle Scholar
- Shaikh, A. A. (2013). Mobile banking adoption issues in Pakistan and challenges ahead. Journal Institute Bankers Pakistan, 80(3), 12–15. Google Scholar
- Shaikh, A. A., & Karjaluoto, H. (2015). Mobile Banking adoption: A literature review. Telematics and Informatics, 32, 129–142. ArticleGoogle Scholar
- Sharma, S. K., Joshi, A., & Sharma, H. (2016). A multi-analytical approach to predict the Facebook usage in higher education. Computers in Human Behavior, 55, 340–353. ArticleGoogle Scholar
- Shmueli, G., & Koppius, O. R. (2011). Predictive analytics in information systems research. MIS Quarterly, 35, 553–572. ArticleGoogle Scholar
- Sim, J. J., Tan, G. W. H., Wong, J. C., Ooi, K. B., & Hew, T. S. (2014). Understanding and predicting the motivators of mobile music acceptance–a multi-stage MRA-artificial neural network approach. Telematics and Informatics, 31(4), 569–584. ArticleGoogle Scholar
- Sinha, P. K. (2003). Shopping orientation in the evolving India market. The Journal of Decision Makers, 28(2), 13–22. ArticleGoogle Scholar
- Sripalawat, J., Thongmak, M., & Ngramyarn, A. (2011). M-banking in metropolitan Bangkok and a comparison with other countries. Journal of Computer Information System, 51(3), 67–76. Google Scholar
- Suoranta, M., & Mattila, M. (2004). Mobile banking and consumer behavior: New insights into the diffusion pattern. Journal of Financial Services Marketing, 8(4), 354–366. ArticleGoogle Scholar
- Tam, C., & Oliveira, T. (2016). Understanding the impact of m-banking on individual performance: DeLone & McLean and TTF perspective. Computers in Human Behavior, 61, 233–244. ArticleGoogle Scholar
- Telecommunication Regulatory Authority Report (2016). https://www.tra.gov.om/pdf/q2-2016.pdf. Accessed on 03 Nov 2016.
- TOO (2016). http://timesofoman.com/article/84406/Business/Oman's-banking-sector-achieves-robust-growth-despite-oil-price-slump. Accessed 03 Nov 2016.
- Vansteenkiste, M., Neyrinck, B., Niemiec, C. P., Soenens, B., Witte, H., & Broeck, A. (2007). On the relations among work value orientations, psychological need satisfaction and job outcomes: A self-determination theory approach. Journal of Occupational and Organizational Psychology, 80(2), 251–277. ArticleGoogle Scholar
- Venkatesh, V., & Davis, F. D. (2000). A theoretical extension of the technology acceptance model: Four longitudinal field studies. Management Science, 46(2), 186–204. ArticleGoogle Scholar
- Venkatesh, V., Morris, M. G., Davis, G. B., & Davis, F. D. (2003). User acceptance of information technology: Toward a unified view. MIS Quarterly, 27, 425–478. ArticleGoogle Scholar
- Wang, Y. M., & Elhag, T. M. (2007). A comparison of neural network, evidential reasoning and multiple regression analysis in modelling bridge risks. Expert Systems with Applications, 32(2), 336–348. ArticleGoogle Scholar
- Wang, Y. S., Wu, M. C., & Wang, H. Y. (2009). Investigating the determinants and age and gender differences in the acceptance of mobile learning. British journal of educational technology, 40(1), 92–118.
- Wessels, L., & Drennan, J. (2010). An investigation of consumer acceptance of M- banking. International Journal of Bank Marketing, 28(7), 547–568. ArticleGoogle Scholar
- Yadav, R., Sharma, S. K., & Tarhini, A. (2016). A multi-analytical approach to understand and predict the mobile commerce adoption. Journal of Enterprise Information Management, 29(2), 222–237. ArticleGoogle Scholar
- Yu, T. K., & Fang, K. (2009). Measuring the post-adoption customer perception of mobile banking services. Cyberpsychology & Behavior, 12(1), 33–35. ArticleGoogle Scholar
- Zhang, L., Zhu, J., & Liu, Q. (2012). A meta-analysis of mobile commerce adoption and the moderating effect of culture. Computers in Human Behavior, 28(5), 1902–1911. ArticleGoogle Scholar
- Zhou, T. (2011). An empirical examination of initial trust in mobile banking. Internet Research, 21(5), 527–540. ArticleGoogle Scholar
- Zhou, T. (2012). Understanding users’ initial trust in mobile banking: An elaboration likelihood perspective. Computers in Human Behavior, 28, 1518–1525. ArticleGoogle Scholar
- Zhou, M. (2016). Chinese university students' acceptance of MOOCs: A self-determination perspective. Computers & Education, 92, 194–203. ArticleGoogle Scholar
Author information
Authors and Affiliations
- Department of Operations Management and Business Statistics, Sultan Qaboos University, Muscat, Oman Sujeet Kumar Sharma
- Sujeet Kumar Sharma